How to Answer AI’s Most Important Question for Enterprises
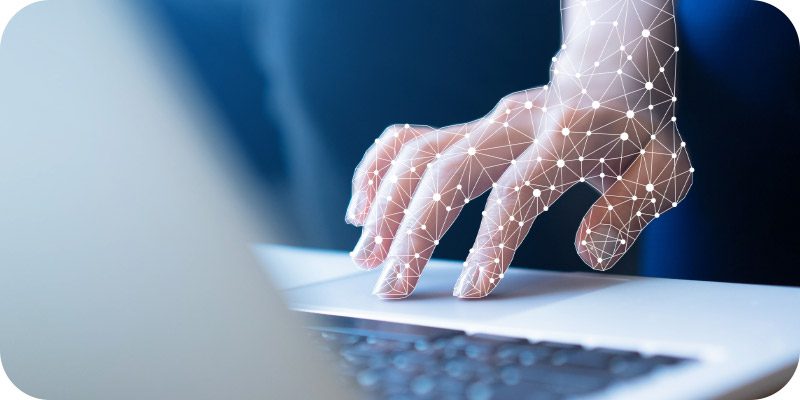
Solutions Review’s Contributed Content Series is a collection of contributed articles written by thought leaders in enterprise tech. In this feature, Commvault‘s Senior Director of Products Pavan Bedadala offers commentary on how to answer AI’s most important question for enterprises.
“We’ve got the data. What’s next?”
It may be the business world’s most important AI question right now, and it’s one nearly every business leader is asking.
Over the last decade, the explosion of digital technologies turbocharged corporate efforts to gather information on customers and internal operations. And now, companies are awash with data that largely sits idle. But that is quickly changing.
ChatGPT and other forms of generative AI have everyone paying attention to the technology. And businesses see an opportunity to finally start making fuller use of their data troves. They want to build their own large language models (LLMs) for such purposes as helping employees with their work, unlocking the power of predictive intelligence to improve decision-making and pin-pointing areas of inefficiency in the business — to cite just a few of the potential applications of the technology.
But such initiatives will require companies to think more critically about their data. The age of simply gathering information just to have it is over. Now, it’s time to put that data to work. And yet, that opens up a host of new challenges that organizational leaders must consider, including how to effectively safeguard all that important corporate information now flowing around the business.
The following four questions provide an initial checklist that executives can use to make sure they are starting their AI pivot on secure footing.
What Information Will We Need to Train the Model?
The first step is figuring out what information is going to fuel the new AI applications. That decision will have a profound impact on future results.
For example, GitHub Co-Pilot is successful because it has vast repositories of code, used by professionals around the world to build applications, at its training disposal.
On the enterprise level, that could mean making sure an internal employee chatbot has access to the handbook so it can answer basic workplace questions. Or, for a manufacturer, it could be funneling all logistics data to one model so it can accurately optimize shipping routes.
Ultimately, organizations may have to broaden their definition of data. The last decade of business intelligence largely focused on using highly-curated information – like financial data. But increasingly, companies can make use of so-called raw data – like video or social media feeds – which are less “clean” but still important to training AI models.
How Do I Gather It?
The world of IT is now hybrid, with some data stored on premises, some in the cloud and, increasingly, some on the various mobile edges of the network – or what the industry refers to as edge computing.
Because information stored in all those locations is often in proprietary formats, gathering all the relevant data becomes much harder. It’s why businesses should work with technology partners that can break down those silos and enable companies to pin-point information using basic commands. Then, those vendors should help customers build secure pipelines so the data can be routed to the right location.
Lastly, the enterprise should adopt access policies that make sure employees are using only the information they are authorized to. A sales leader, for example, probably doesn’t need to be able to view employee social security numbers. Such a policy can actually end up saving organizations money, as it helps make sure that data is being stored in the most efficient manner.
Why Do We Need Real-Time Data?
Organizations must then make sure they are constantly collecting relevant data and funneling it to the appropriate AI system.
Most organizations are accustomed to an environment where, over several hours, information could be gathered, cleaned and transported to the proper repository for analysis. Such a delay wasn’t particularly disruptive, as many of the projects focused on providing a historical view of the business – like gathering yesterday’s sales totals.
AI, however, requires a constant ingestion of data from the relevant sources so the model is always learning and improving. Businesses, moreover, want to be making decisions based on the most up-to-date information, which means they can’t afford an hours-long delay in getting the data to the right location for processing.
For example, if the goal is to monitor real-time brand sentiment, the AI system will need continuous access to social media feeds. Every post could mean an opportunity for a company to improve its brand reputation, or see it suffer as a result of a customer complaint.
And because the model is constantly analyzing that data, it will get better at picking out the most relevant information so companies can act quicker to address any issues, helping to ultimately drive better customer loyalty.
How Do We Make Sure the AI Model is Working Right?
AI models are living systems. If properly designed and implemented, they get more powerful with each use. If done wrong, the application could produce inaccurate insights that ultimately harm the health of the business – as when Zillow’s algorithm failed to accurately predict home prices.
Companies must continually evaluate the performance of the applications – as well as set realistic and specific expectations for what the LLMs can achieve. And they must constantly monitor for potential new data sources that may help improve outcomes.
For example, in an effort to become more efficient, the company may decide to install internet-enabled sensors around their manufacturing plant. The company would then have new information that can be critical in helping cut down on energy costs. But if that data isn’t routed to the right location, the business would have trouble getting the benefit from those investments. AI could help determine the optimal routing.
The journey to AI can be long, confusing and frustrating, but the outcomes are well worth it. And getting the foundation right now is vital to success later. By devoting time to considering these four important steps, businesses can eliminate many of the headaches that often arise, get value from the systems quicker and ultimately be more prepared to take advantage of the coming decade of AI advancement.
Most importantly, you’ll have to answer the next question: “Now what?”