Decades ago, most of us probably had a slightly different vision of how autonomous driving (AD) would look by now: robots behind the wheel; quiet, sleek cars quietly zipping everyone to their destinations; and maybe even the end of traffic. While we’re clearly making progress and getting there (except for maybe the robots behind the wheel part), it hasn’t necessarily been a smooth road (pun intended).
In some ways, 2023 was a setback year for AD. McKinsey notes that previously optimistic delivery timelines have been pushed back, with fully autonomous (i.e., no human driver in the car) robo-taxis now likely to appear widely by 2030, a slip of several years. Autonomous trucking may come a few years earlier.
While some manufacturers have scaled back or even stopped AD initiatives, others are pushing forward, incorporating new artificial intelligence (AI) technologies. One of those is automobile software innovator Ghost Autonomy.
Recently, Pure Storage Field CTO Michael Cornwell sat down with Ghost Autonomy’s founder and CEO, John Hayes — who also, incidentally, founded Pure Storage — to chat about the present and future of AD.
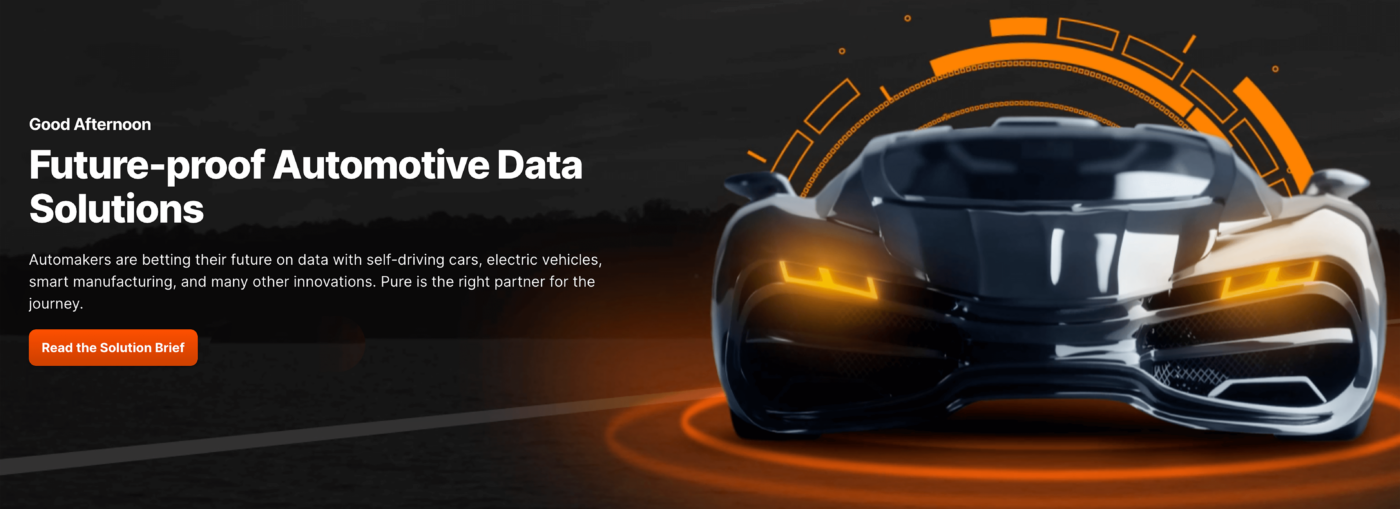
Ghost Autonomy and LLM Innovation
Ghost Autonomy provides a complete AD software stack that can be used with a wide range of hardware components (cameras, radar, sensors, etc.). Functionality is customizable because not all vehicles are alike: A sedan drives differently than an SUV.
Everything is API-enabled, and new features are easily deployed via over-the-air updates.
Ghost is moving ahead in the industry by incorporating AI via large language models (LLMs) and, in particular, what are known as multimodal large language models (MLLMs). These go beyond learning from text and add image and video inputs, precisely the kinds of things you’d need to “understand” the world surrounding a moving vehicle.
An MLLM is capable of reasoning and making decisions based on a multitude of factors. For example, a common driving scenario is having to navigate a construction zone. This can introduce many obstacles into the driving experience, such as a lane closure, multiple traffic cones, construction equipment, a worker holding a stop/go sign, and so on, in addition to the usual collection of other vehicles and pedestrians. It’s not enough to identify the individual items. The MLLM has to put together a true image of the complete situation. This is the kind of thing human brains are expert at, but it’s a major challenge in autonomous driving.
The AD Tech Stack: Hardware, Software, LLMs and Data
Nevertheless, it’s one Ghost is facing head-on.
“Autonomy is undergoing a radical transformation.” John said. “People used to think of autonomy as primarily a hardware problem. What we’re seeing today is almost every company has come to realize it’s predominantly both a software challenge and a data challenge to make it work.”
LLMs are a key ingredient for AD success, he added.
“LLMs can be an essential part of the online driving stack, where you have a system in a car and then you have a dramatically smarter model in the data center that supervises that based on the entire scene,” John said.
AI Acceleration: More Gas, Less Brake
One hump AI in general is still trying to get over is speed. John admitted it’s still pretty slow, but there are two phases:
- Getting reliable answers
- Operationalizing those reliable answers
At the moment, we’re still transitioning into phase 2, he said, and the motivation for speed isn’t just for cars but other key AI initiatives, such as augmented reality. “Our expectation is that these models will get faster,” John said. “Also you can customize the infrastructure to have better parallelism.”
In autonomous driving, the next step will be adding environmental “context” through the increased incorporation of media (such as video), John said.
Delivering on Autonomous Safety
Developing and delivering on AD safety has also been a slow, winding road. John explained that AD safety requires a different approach from traditional automotive safety.
“A lot of safety was done through very very careful detailed analysis of the designs themselves,” he said. “With AI, you don’t reason about it in the same way. So what you actually end up doing is measuring the performance. In this case, it’s a continuous system where you’re always collecting new data and you’re matching up whether the performance of the real world with your model matches the performance that you got with your data set.”
This shifts the problem away from one of the logical capacity of the engineers, John said, to one of managing a data set to add more and more subtle variations that are eventually representative of the real world.
“This tends to be an iterative process and it continues past shipping,” he said. “And so you have to bring a lot of parts of your organization together to make this work because often you’re defining the product as you go along by the performance of your AI and trying to make a specific promise to your customer about how it works and when it works based on the numbers that you’ve measured so far, with the expectation that you’re going to improve that over time and change the product over time.”
Hear More from the Ghost Autonomy Founder John Hayes
Watch the entire conversation on-demand, “Accelerating the AI Development Data Pipeline for Autonomous, Software-defined Vehicles” to learn more about the AD software development lifecycle and the challenges of becoming a software engineering leader in the AD space.
Watch the webinar on demand.